
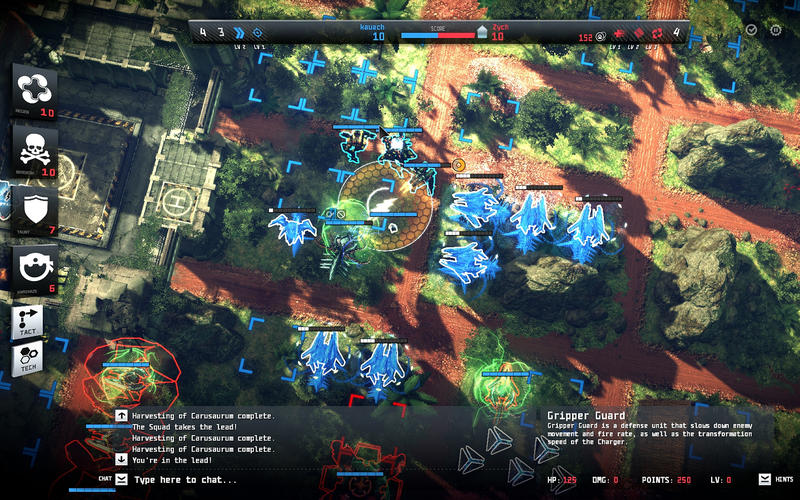
While end-to-end learning has demonstrated success in many machine learning problems, including deep learning algorithm designs, such an approach for deep one-class classifiers often suffer from degeneration in which the model outputs the same results regardless of the input. The framework achieves a new state-of-the-art on the MVTec benchmark.Ī Two-Stage Framework for Deep One-Class Classification

We then follow up on this in “ CutPaste: Self-Supervised Learning for Anomaly Detection and Localization”, presented at CVPR 2021, in which we propose a new representation learning algorithm under the same framework for a realistic industrial defect detection problem. The algorithm is simple to train and results in state-of-the-art performance on various benchmarks, including CIFAR, f-MNIST, Cat vs Dog and CelebA. In “ Learning and Evaluating Representations for Deep One-class Classification”, presented at ICLR 2021, we outline a 2-stage framework that makes use of recent progress on self-supervised representation learning and classic one-class algorithms. As such, combining one-class classifiers with these recent successes in deep representation learning is an under-explored opportunity for the detection of anomalous data. On the other hand, substantial progress has been made in learning visual representations from unlabeled data via self-supervised learning, including rotation prediction and contrastive learning. Unfortunately, these classical algorithms do not benefit from the representation learning that makes machine learning so powerful.
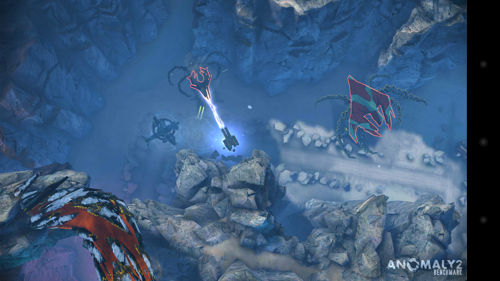
As such, one-class classification, such as one-class support vector machine (OC-SVM) or support vector data description (SVDD), is particularly relevant to anomaly detection because it assumes the training data are all normal examples, and aims to identify whether an example belongs to the same distribution as the training data. It is most often used when it is easy to collect a large amount of known-normal examples but where anomalous data is rare and difficult to find. Posted by Chun-Liang Li and Kihyuk Sohn, Research Scientists, Google CloudĪnomaly detection (sometimes called outlier detection or out-of-distribution detection) is one of the most common machine learning applications across many domains, from defect detection in manufacturing to fraudulent transaction detection in finance.
